If you have ever attempted to develop your own machine learning models or algorithms, or are planning to do so, you may have encountered a common obstacle: acquiring enough data that is diverse enough to effectively train your model.
Fortunately, synthetic data offers a solution to this problem.
As AI projects become more prevalent, the need for synthetic data generation is becoming increasingly apparent. In fact, a study by Gartner predicts that by 2024, 60% of the data used for the development of AI and analytics projects will be synthetically generated. This highlights the importance of synthetic data in training AI models efficiently and effectively.
In this blog post, we’ll explain synthetic data, why you need to use it, the benefits of using synthetic data over real-world data, and important use cases.
Let’s begin!
What is synthetic data?
Synthetic data refers to artificially generated data that simulates real-world data without revealing any sensitive or confidential information. The process of creating synthetic data involves using statistical methods and machine learning algorithms to generate data that mimics the distribution, patterns, and correlations found in real-world data.
Synthetic data is a useful tool for testing and validating machine learning models, as it can be used to create large datasets that represent a range of scenarios and edge cases.
Let’s continue by understanding why synthetic data is so necessary.
Why do you need synthetic data?
In today’s data-driven world, data is a valuable resource for organizations to make informed decisions. However, collecting, labeling, and cleaning data can be expensive and time-consuming. Furthermore, companies may not have access to sufficient data or face privacy concerns. That’s why synthetic data can be essential for businesses in such situations.
Below, we list the most important reasons why you need synthetic data:
- Mitigate data privacy risks
- Lack of real-world data
- Effortlessly generate large amounts of data
Mitigating data privacy risks
Businesses often require synthetic data to minimize data privacy risks. Collecting real-world data can pose challenges in complying with data protection regulations and preserving the privacy of customers and employees. This is particularly the case when working with sensitive data such as medical or financial records. Synthetic data can provide a solution by generating realistic, yet artificial datasets.
Lack of real-world data
Another situation in which synthetic data is useful is when there is a lack of real-world data. In some cases, companies may not have enough data to train a machine-learning model effectively. For example, if a company is developing a new model, there may not be enough historical data to train it.
Effortlessly generate large amounts of data
Generating significant amounts of artificial datasets in a short period is a straightforward process, which makes it a valuable asset for organizations seeking to quickly enhance and train their machine learning models. Synthetic data provides an ideal solution for overcoming the limitations of scarce data availability.
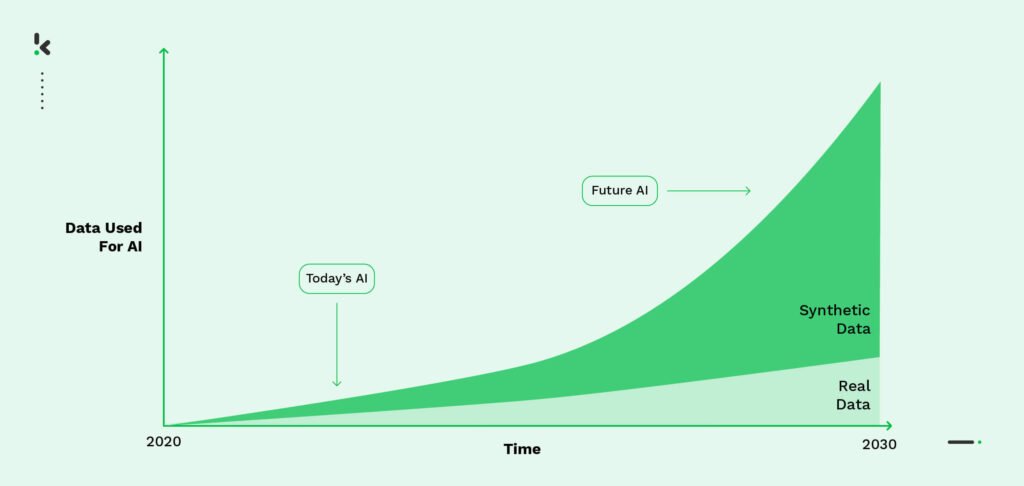
Now that you are aware of the reasons why synthetic data is necessary, let’s delve into the advantages it offers over real-world data, as well as any potential drawbacks.
Synthetic Data vs. Real Data
When it comes to choosing which type of data you should use, it’s important to understand both the advantages as well as any potential disadvantages.
Let’s start by examining the potential drawbacks.
The quality of synthetic data may be inferior
Synthetic data may be of inferior quality compared to real data, particularly in complex or multi-variable scenarios. Algorithms used to generate synthetic data may not capture the full complexity and nuance of real-world data, leading to inaccuracies and biases that can impact analysis and modeling accuracy.
Synthetic data may not represent the full range of real data
Synthetic data may not cover the full range of real data, missing rare events or outlier data points. This can lead to overfitting and poor generalization of analysis or modeling based on synthetic data, despite mimicking certain patterns or distributions.
The use of synthetic data raises ethical concerns
Synthetic data can raise ethical concerns, especially if it replaces real-world data that inform important decisions or policies. Although synthetic data can be useful in scarcity or challenging data acquisition scenarios, it should not replace real data when it’s available and appropriate to use. The transparency and accountability of synthetic data generation may also be challenging to determine, making it harder for stakeholders to trust analysis or modeling based on it.
Let us now investigate the advantages of utilizing synthetic data.
Cost-effective
Synthetic data can be generated at a lower cost than real-world data, which benefits small to medium-sized businesses that lack the budget for extensive data collection. This allows them to save money while still being able to train AI models effectively.
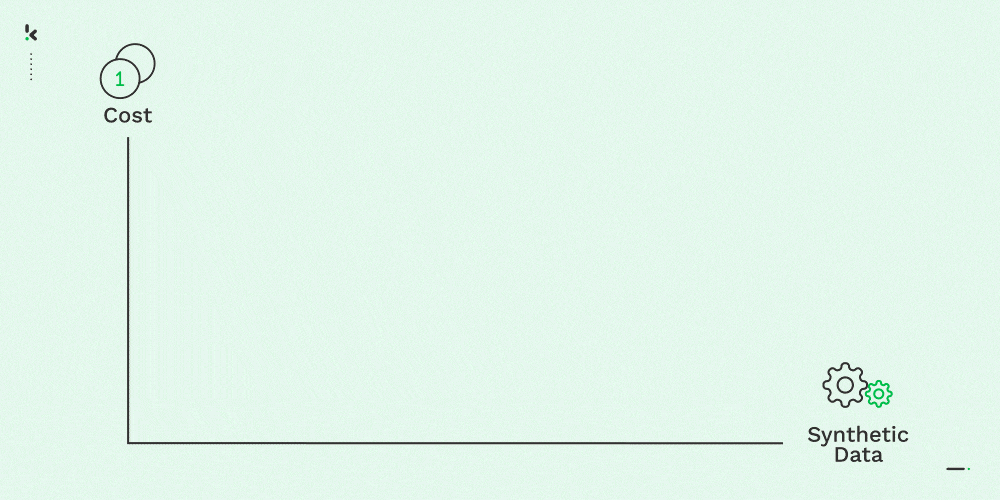
Privacy protection
As previously mentioned, synthetic data is generated artificially and does not contain any sensitive information that could potentially infringe upon regulations, making it a safer option for businesses.
Flexibility and control
Synthetic data offers more flexibility and control over data than real-world data. Companies can customize synthetic datasets to meet their specific needs, including manipulating variables and parameters to generate different scenarios and test various hypotheses.
Reduced bias
While the use of synthetic data can sometimes introduce unwanted biases into datasets, it can also play a valuable role in reducing biases in environments that require modifications. Synthetic data can be generated with controlled parameters and known characteristics, which allows for the reduction or elimination of biases present in real-world data.
Additionally, synthetic datasets can be used to address imbalanced datasets by providing a more balanced distribution of data, and can simulate scenarios that are difficult to capture in real-world settings, thereby producing more diverse and representative data for model training.
To overcome any potential drawbacks of using only synthetic datasets in training AI models, one possible solution is to combine synthetic data with real-world data. This can lead to better performance and more robust models.
Types of synthetic data
After examining the potential benefits and limitations of using synthetic data compared to real-world data, it’s time to explore the various types of synthetic data:
- Synthetic text
- Synthetic media
- Synthetic tabular data
Synthetic text
Synthetic text mimics real-world text data, created with Natural Language Processing (NLP) techniques like language models and deep learning models. It’s beneficial for developing chatbots such as ChatGPT, translation systems, and sentiment analysis tools. Additionally, data augmentation can be done by adding artificial text to existing datasets to improve machine learning model quality.
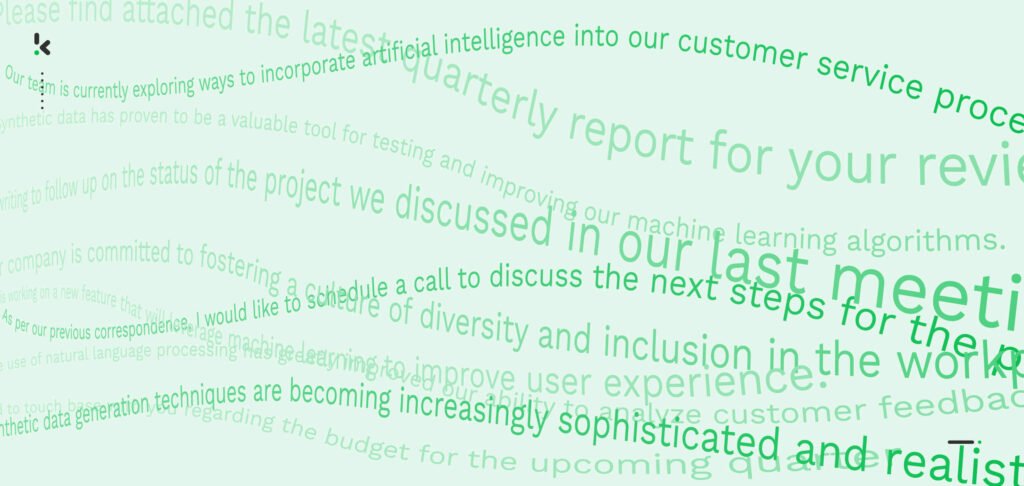
Synthetic media
Synthetic media is computer-generated media that resembles real-world images, videos, and audio, created with advanced techniques such as computer graphics and deep learning models. It has many applications, including content creation, virtual reality, and simulation, with potential uses in movies, virtual assistants, and music production. It’s a versatile tool for solving various challenges in different fields.
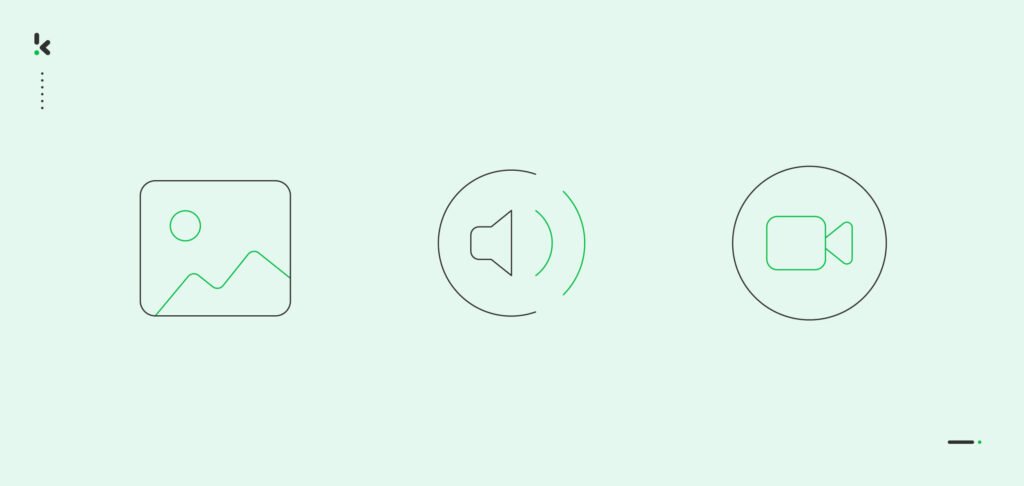
Synthetic tabular data
Synthetic tabular data imitate real-world data in table form, created with statistical models like decision trees and random forests. It’s useful for data augmentation, data masking, and data sharing, where synthetic data is added to existing datasets to increase their size and variety or to retain statistical properties while preserving sensitive information.
Synthetic data use cases
Now that you have gained an understanding of what synthetic data is and how it differs from real-world data, let’s explore some important use cases for synthetic data for different industries, including:
- Healthcare
- Finance
- Automotive
- Retail
- Manufacturing
Healthcare
In healthcare, synthetic data has the potential to train machine learning models that can effectively diagnose diseases and detect health hazards. This can be highly beneficial in circumstances where obtaining genuine patient data is challenging due to factors such as privacy concerns or availability.
Finance
Synthetic data has the capacity to train machine learning models that can recognize patterns and forecast market trends. This can enable financial institutions to make better-informed investment decisions and more efficiently manage risks.
Automotive
By utilizing synthetic data, it is possible to train deep learning models that are capable of identifying and categorizing various objects on the road, including pedestrians and other vehicles. This has immense potential in the development of self-driving vehicles that can safely navigate through intricate environments.
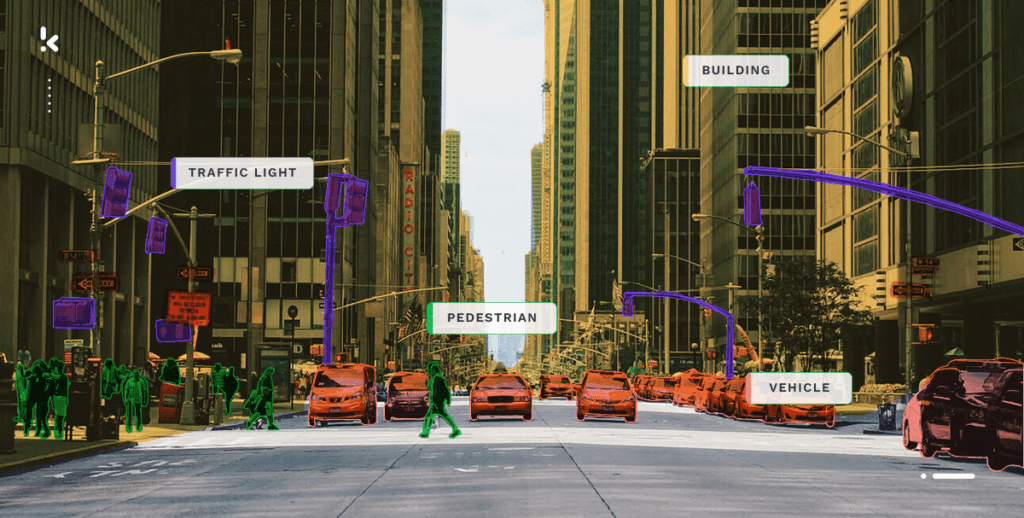
Retail
Within the retail sector, synthetic data is capable of generating realistic simulations of customer behavior and preferences, providing retailers with insights to optimize their marketing and sales strategies. For instance, synthetic data can predict the popularity of products among specific demographics or during particular times of the year.
Manufacturing
Synthetic data has the potential to train machine learning models that can recognize patterns in manufacturing processes and forecast equipment failures. This can significantly reduce downtime and enhance the overall efficiency of manufacturers.
Get started with using synthetic data
By now we hopefully proved our point that synthetic data in many cases is a more efficient and cost-effective solution than using real data. If you’re interested in exploring the potential of synthetic data, Klippa DataNorth is your go-to partner. We specialize in generating high-quality synthetic datasets that are tailored to your unique needs, enabling you to conduct AI model training with confidence.
Our team of experts is dedicated to helping companies and organizations improve their AI model performance by providing large volumes of synthetic data for training purposes. Whether you need synthetic data for compliance or AI model training, we’re here to assist you in harnessing the benefits of synthetic data.
Our services include custom synthetic data generation, data labeling and annotation, and data validation and testing. Whatever you need, we’ll work closely together with you to deliver synthetic datasets that align with your business objectives, while complying with GDPR regulations.
If you’re ready to leverage the power of synthetic data for your AI model training, contact DataNorth today. Our experienced team is dedicated to helping you achieve success.