In today’s dynamic digital landscape, businesses are constantly looking for innovative technologies to elevate operational efficiency and customer experiences. Among the groundbreaking advancements in Artificial Intelligence, Retrieval-Augmented Generation (RAG) stands out as a new game-changer.
This blog dives into Retrieval Augmented Generation, exploring its mechanisms, benefits, and practical applications for businesses looking for innovative solutions using AI.
So let’s get started!
What is RAG?
Retrieval-Augmented Generation, or RAG for short, is like a super-powered research assistant for your AI systems, enhancing the applicability of large language models by integrating them with external knowledge bases.
So how does it actually work you might wonder? Well, RAG combines the strengths of two key components: a retriever and a generator.
The retriever, acting as a knowledge seeker, pulls relevant information from your database, often stored in vector databases. The generator then leverages this retrieved information to craft accurate and contextually aware responses.
The process begins with a user query. The retriever, like a skilled librarian, receives the user prompt and searches through a large knowledge base, often embedded in vector databases, to locate relevant documents. This retrieved information, combined with pre-trained models, fuels the generator to create a response for the original prompt that is both insightful and reliable.
This dual approach offers several advantages:
- Minimized Error Rates: By responses based on facts from retrieved information, RAG significantly reduces the risk of errors or outdated information.
- Enhanced Contextual Understanding: RAG excels at understanding the nuances of context, crucial for tasks like automated customer support, data analysis or interpreting numerical representations.
- Up-to-date Relevance: By integrating the latest information, RAG ensures responses remain relevant and factually accurate, keeping your business at the forefront of knowledge, without having to retrain a whole AI model.
Overall, this approach enables generative AI systems to produce more accurate, relevant, and contextually aware responses, making them powerful tools for a wide range of applications.
Why use RAG?
Retrieval-Augmented Generation, is a significant advancement in the world of AI, specifically in the field of Natural Language Processing (NLP). By combing the strengths of retrieved-based models and generative models RAG enhances the overal quality and accuracy of generative AI response.
Let’s have a look at some key reasons why you should be using RAG.
- Addressing LLM limitations: Traditional LLMs are limited to the knowledge they were trained on, which can become outdated and generate inaccurate responses. RAG addresses this by allowing LLMs to access and integrate up-to-date information from external sources embedded in vector databases, ensuring relevant responses are current and accurate
- Reducing hallucinatons: LLMs can sometimes generate plausible but incorrect information, known as hallucinations. RAG mitigates this by grounding responses in factual, retrieved data, thereby enhancing the reliability and trustworthiness of the outputs
- Cost-effectiveness: RAG allows for the integration of new information without the need to retrain the entire model, which can be both time-consuming and expensive. This makes it a cost-effective solution for maintaining the relevance and accuracy of AI systems
- Versatility and adaptability: RAG can be applied across various domains and industries, from customer service and healthcare to finance and media. Its ability to provide contextually relevant and accurate information makes it a versatile tool for enhancing AI applications
- Enhancing user trust: By providing sources for the information used in responses, RAG builds user trust. Users can verify the accuracy of the information, enhancing the user experience. This is particularly important in fields requiring high reliability, such as legal and medical applications.
Why is RAG so popular?
RAG’s ability to create more accurate responses and increase the relevance and trustworthiness of AI-generated responses, combined with its cost-effectiveness and versatility, makes it a popular choice for improving the performance of LLMs. Its adoption by major companies and application across various domains underscore its potential to revolutionize how we use generative AI.
Now that you know what RAG is and why it has become so popular, let’s look at how you can leverage RAG to enhance operations in various parts of your organization.
Transforming Businesses: Benefits of RAG
RAG offers some transformative benefits across various parts of your organization:
Improving Customer Service Accuracy
Customer service bots significantly enhance accuracy through the use of RAG. By accessing real-time databases, these bots deliver timely, relevant, and personalized responses, boosting customer satisfaction and fostering trust in your service capabilities. Research indicates that 93% of customers stay loyal to companies offering excellent support, driving businesses to continually seek better service strategies.
Streamlining Content Personalization
For content-driven businesses, RAG is a game-changer. Content personalization, based on user data and behavior, leads to increased engagement rates. Whether for marketing campaigns, product recommendations, or online interactions, RAG empowers you to create content that fits your audience.
Minimizing Errors and Enhancing Decision-Making
By utilizing big datasets, RAG minimizes errors in data processing and output generation, crucial in fields demanding high precision, such as finance and healthcare. The enhanced decision-making support provided by RAG paves the way for better business outcomes and operational efficiency.
Operational Efficiency through Streamlined Data Handling
Implementing RAG facilitates efficient data handling through automated processes, reducing the workload on human resources and minimizing errors. This improved process efficiency translates into significant cost savings and faster response times across various business operations.
Implementing RAG: A Practical Roadmap
Embrace the power of RAG to revolutionize your business operations and customer interactions. Here’s a step-by-step guide to successful RAG implementation:
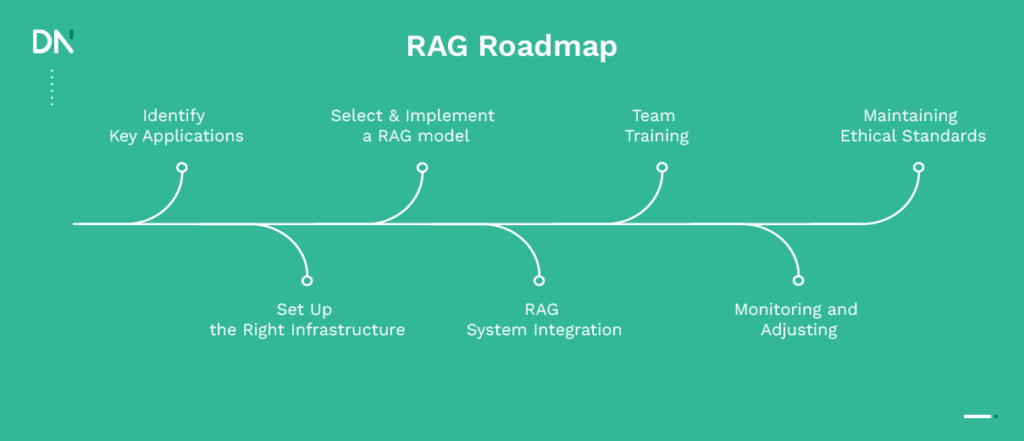
1. Identifying Key Applications
Assess where RAG can deliver the most value. Common applications include:
- Customer Service: Elevate chatbot capabilities by integrating RAG to provide accurate and contextually relevant answers based on past interactions.
- Content Creation: Utilize RAG for dynamic content generation, where the system retrieves and synthesizes information to produce unique, tailored content.
2. Setting Up the Right Infrastructure
Ensure your infrastructure can support RAG by upgrading to:
- High-Performance Servers: Essential for processing large datasets and running complex models efficiently.
- Advanced Data Management Systems: To effectively handle and retrieve data required for RAG operations.
3. Selecting and Implementing a RAG Model
Choose a RAG model that aligns with your specific needs. Consider options like:
- Dense Passage Retrieval (DPR): Ideal for scenarios requiring precise information retrieval from large text corpora, such as legal or medical research.
- Latent Retrieval for Text Generation: Perfect for applications needing creative and high-quality text outputs, like marketing content creation.
4. Integrating RAG into Your Systems
Plan the integration carefully:
- API Integration: Seamlessly connect RAG capabilities with your existing systems using APIs for efficient data exchange.
- Workflow Alignment: Adjust and refine your business workflows to incorporate RAG functionalities without disrupting current operations.
5. Training Your Team
Equip your team with the knowledge to utilize the new tools effectively:
- Technical Training: Provide detailed sessions on the technical aspects of RAG.
- Operational Training: Teach staff how RAG fits into their daily tasks and how it can simplify and enhance their work.
6. Monitoring and Adjusting
Implement monitoring tools to track the effectiveness of RAG:
- Performance Metrics: Regularly review metrics to assess how well the RAG model meets business goals.
- Feedback Loops: Utilize feedback from both users and the system itself to refine and optimize the RAG implementation.
7. Maintaining Ethical Standards
Ensure your RAG deployment adheres to ethical AI guidelines:
- Transparency: Keep stakeholders informed about how RAG technologies are applied within your business.
- Data Privacy: Implement strict protocols to protect the data used and generated by your RAG systems.
Industry Case Studies: RAG in Action
Let’s explore how diverse industries are leveraging RAG to achieve remarkable results:
Online Retail: Boosting Sales and Engagement
A major online retailer implemented RAG to personalize customer interactions, leading to:
- 30% surge in customer engagement: By tailoring product recommendations and offers to individual preferences, RAG created a more engaging and relevant shopping experience.
- 20% increase in sales conversions: The personalized approach powered by RAG resulted in higher conversion rates, driving revenue growth for the retailer.
Financial Services: Enhancing Customer Experience
A global banking institution integrated RAG into their customer service interactions, resulting in:
- Faster response times to customer queries: RAG enabled the bank to provide swift and accurate answers to customer inquiries, improving overall satisfaction.
- Higher accuracy in financial advice: RAG-powered systems offered more reliable and personalized financial guidance, fostering trust and loyalty among customers.
Healthcare: Streamlining Patient Care
A healthcare provider incorporated RAG into their clinical decision support systems, achieving:
- Reduced diagnostic times: RAG facilitated faster and more accurate diagnoses by providing healthcare professionals with relevant medical information and insights.
- Improved patient outcomes: The enhanced decision-making support provided by RAG led to better treatment plans and ultimately, improved patient outcomes.
Media: Tailoring Content for Enhanced Engagement
A leading media company utilizes RAG to generate personalized news content, leading to:
- Increased reader engagement: By delivering news stories aligned with individual interests, RAG fostered deeper engagement and a more loyal readership.
- Higher subscription rates: The personalized content experience powered by RAG attracted more subscribers, contributing to the media company’s growth.
Final Thoughts: Embracing the Future with RAG
As technology advances, integrating sophisticated tools like RAG becomes increasingly crucial for businesses seeking transformative growth and sustainability in a competitive market.
RAG empowers businesses to:
- Stay Relevant: By keeping pace with the latest information and trends, RAG ensures your business remains at the forefront of knowledge.
- Become More Responsive: RAG enables you to address customer needs and market demands with agility and precision.
- Build Resilience: By enhancing decision-making and operational efficiency, RAG equips your business to navigate challenges and thrive in a dynamic environment.
The future belongs to those who innovate. Embrace RAG, innovate relentlessly, and unlock the potential for a more successful and sustainable future for your business.
Need help implementing RAG in your existing AI implementations or do you have a Use Case in mind that makes use of the power of RAG? With the AI Development & Implementation services of DataNorth we can help you achieve your goals and make a future-proof organization.